Oct 27, 2021
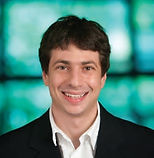
by Dr. Aaron Roth
Data Professor, Department of Computer and Information Science
University of Pennsylvania
Bio
Aaron Roth is a professor of Computer and Information Sciences at the University of Pennsylvania, affiliated with the Warren Center for Network and Data Science, and co-director of the Networked and Social Systems Engineering (NETS) program. He is also an Amazon Scholar at Amazon AWS. He is the recipient of a Presidential Early Career Award for Scientists and Engineers (PECASE) awarded by President Obama in 2016, an Alfred P. Sloan Research Fellowship, and an NSF CAREER award. His research focuses on the algorithmic foundations of data privacy, algorithmic fairness, game theory, learning theory, and machine learning. Together with Cynthia Dwork, he is the author of the book “The Algorithmic Foundations of Differential Privacy.” Together with Michael Kearns, he is the author of “The Ethical Algorithm”.
Abstract
How can we quantify the accuracy and uncertainty of predictions that we make in online decision problems? Standard approaches, like asking for calibrated predictions or giving prediction intervals using conformal methods give marginal guarantees (i.e. they offer promises that are averages over the history of data points. Guarantees like this are unsatisfying when the data points correspond to people, and the predictions are used in important contexts) like personalized medicine.
In this work, we study how to give stronger than marginal ("multivalid") guarantees for estimates of means, moments, and prediction intervals. Guarantees like this are valid not just as averaged over the entire population, but also as averaged over an enormous number of potentially intersecting demographic groups. We leverage techniques from game theory to give efficient algorithms promising these guarantees even in adversarial environments.