Jan 30, 2023
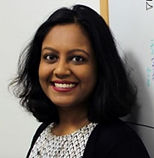
by Dr. Swati Gupta
Fouts Family Early Career Professor and Assistant Professor
Georgia Institute of Technology
Bio
Dr. Swati Gupta is a Fouts Family Early Career Professor and Assistant Professor in the Stewart School of Industrial & Systems Engineering at Georgia Tech. She serves as the lead of Ethical AI in the NSF AI Institute on Advances in Optimization. She received a Ph.D. in Operations Research from MIT. Her research interests include optimization, machine learning, and algorithmic fairness. She received the Class of 1934: Student Recognition of Excellence in Teaching in 2021 and 2022 at Georgia Tech, the JP Morgan Early Career Faculty Award in 2021, and the NSF CISE Research Initiation Initiative Award in 2019, and the Google Women in Engineering Award (India) in 2011. She was also awarded the prestigious Simons-Berkeley Research Fellowship in 2017-2018, where she was selected as the Microsoft Research Fellow in 2018. Her research and students have received recognition at various venues like INFORMS Doing Good with OR 2022 (finalist), MIP Poster 2022 (honorable mention), INFORMS Undergraduate Operations Research 2018 (honorable mention), INFORMS Computing Society 2016 (special recognition), and INFORMS Service Science Student Paper 2016 (finalist). Dr. Gupta’s research is partially funded by the NSF and DARPA.
Abstract
Optimization and statistical models based on historical and socio-economic data that do not incorporate fairness desiderata can lead to unfair, discriminatory, or biased outcomes. New ideas are needed to ensure that the developed systems are accountable under uncertainty and reduce a deeper propagation of biases in multi-level decisions. In this talk, I will first discuss methods for ensuring ethical hiring. Recent run-ins of Microsoft and Well Fargo with the Labor Department’s OFCCP highlight a paradox: failing to address workforce imbalance can result in legal sanctions and scrutiny, but proactive measures to address these issues might result in the same legal conflict. I will propose that partially-ordered sets, “posets”, can be used to transparently account for known uncertainties and biases in evaluation data, giving rise to an interesting class of optimization problems. We will showcase how to ensure a “competitive” online selection of candidates with this model. Keeping in mind the requirements of U.S. anti-discrimination law, however, certain methods can be construed as illegal (e.g., imposing quotas). I will discuss the tensions with the law and ways to argue the legal feasibility of our proposed approach. I will also briefly discuss ethical decision-making in other applications such as admissions, detection of critical diseases, facility location, and gerrymandering.
Date: Monday, January 30, 2023
Time: 2:00 – 3:00 p.m. US Central Time
Location: Blocker 220 (in person)
Zoom: 998 4499 3279 (ID) & 724615 (PWD)