Feb 23, 2022
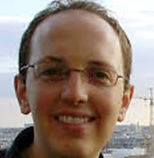
by Dr. Brandon Booth
Postdoctoral Research Associate, Emotive Computing Lab
University of Colorado Boulder
Bio
Brandon Booth is a postdoctoral research associate at CU Boulder working in the Emotive Computing Lab. His research focuses on using multi-modal machine learning techniques to model human perception, behavior, and experiences and developing algorithms to reduce the impact of inadvertent human biases and errors. He received his Ph.D. in computer science from the University of Southern California, and his work on continuous annotation fusion won the ACM AVEC gold-standard emotion representation challenge. He has a diverse industry background developing video games, serious games, robots, computer vision, and human-computer interaction systems.
Abstract
Modern machine learning has become a powerful tool for modeling human preferences and is being increasingly used to aid in decision making that affects ordinary people. In low-stakes domains such as advertising or recommendation systems, differences in the quality of the AI-aided decisions are relatively innocuous, however, the presence of systemic differences in accuracy can have profound impacts on the opportunities and affordances provided to different groups of people when AI is used to make decisions in high-stakes settings, such as loan approval or pre-employment screening. This issue is of primary concern in the field of procedural justice, which aims to understand and mitigate the impact of systemic biases in AI assessment systems. In this talk, I will introduce the concepts of bias and fairness from a psychometric perspective and discuss the primary challenges to reducing bias and achieving fairness in the context of machine learning for human assessment. We will explore emerging methods and conceptual techniques for identifying bias in machine learning and constructing sound arguments for stakeholder-defined fairness goals. I will demonstrate how to measure some types of bias and assess fairness in AI in a case study of "automated video interviews" where legal constraints such as the US Civil Rights Act are important concerns. We will examine the effectiveness of some bias mitigation strategies and discuss important future considerations for addressing bias and fairness concerns in AI systems that ultimately will need to function at a societal scale.